Do you know that feeling? You hear about a concept or tool like “Markov Chains”. A few days later, you hear it mentioned again. And then again. Everybody talks about it and you get curious. This happened to me the past few months. Last week, I finally gave into my curiosity and checked out
Markov Chains. I was very surprised: I realized that any simulation modeller would know Markov Chains already as it is a (very small) subset of what you can do with agent-based modelling (future blog post in the pipeline on this, rest assured).
Anyhow, a controversial thought crossed my mind and I’d like to color it in a little: What if agent-based modelling would have become the business hype that “Big data” and “Analytics” are today? What would the world look like and how would business differ? What if the
sexiest job of the 21st century were … simulation modeller? Let’s have a think…
Recently, a senior marketing consultant from a very renowned management consultancy told me this story: he worked his *** off to decide if the famous German candy “Kinderschokolade” should get a new face as the traditional one stuck around for decades.
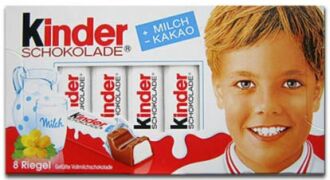
Would you want to change this face?
After months of intense analysis, the final presentation for the CEO was due. He delivered a clear message: “better don’t change”. The CEO listened closely. Then he dropped the bomb: “I still want a new face, let’s do it anyway”…
I said it before and must clarify this again: big data and Analytics are awesome. Fundamentally, they aim to bring objective measures into business decision making, replacing the good old CEO gut feel.
Not only am I glad about big data but I also understand why it (rightfully) became so widespread.
- First, it does deliver business insight and companies get real value from using it.
- It is conceptually easy to understand: you try to obtain insights from large datasets, ideally even combining data that were never analysed together. Methods seem well-established from the statistics community and can be applied with ever-more available computing power
- It is (relatively) easy to get quick results. If you throw your data at a data scientist, they can show you unexpected insights in minutes. Positive management feedback loop engaged
However, there are limits that are largely ignored (for example the implicit assumption that your historical data is representative, see
my discussion here). Moreover, some techniques are re-discoveries of things that have been around for decades or more.
So what if agent-based modelling were the Big Data/Analytics hype? First, I don’t think there is a better/worse or right/wrong fight between either. But let’s imagine a world where agent-based modelling caught on fire 8 years ago and became the big thing everybody talks about. What would business look like?
As mentioned above, I was intrigued to find that Markov Chains are but a small subset of what an agent-based model can do. In our “what-if…” world, people would only model systems based on Markov Chain simplifications if the fast solutions justify it. However, even simple systems often exhibit non-Markov behavior such as “leave a state only after 5 minutes” or “only leave this state if another state tells you to”. These more realistic conditions are trivially easy to implement into an agent-based simulation model. Plus, you could have several agents all following their own part-Markov chains but influence each other, if needed.
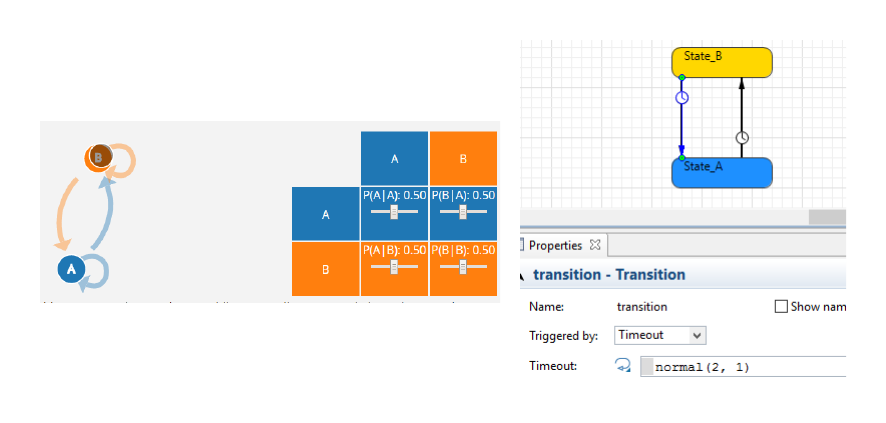
Typical Markov Chain setup (left, http://setosa.io/blog/2014/07/26/markov-chains/) and same setup in an agent-based model (AnyLogic).
As discussed in my blog on post on Analytics and simulation, most Big Data Analytics (of time-dependent processes such as manufacturing, logistics, supply-chains…) implicitly assume that their historical data is a good representation of the past. However, you might have experienced a very unusual past without being aware. Extrapolating from your dataset into the future might be a very bad idea.
In our agent-based world, businesses would simulate their past and then create different alternate pasts to see how (un-)likely their actual past was. Based on that stochastic knowledge, they predict future developments with agent-based models. These use big data, but in a sophisticated way: they are informed by the processes that define a business (how do we handle X? How do we produce Y?…).
In this world, our data scientists are forced to understand the world around them. They still need to crunch big data but it is to inform about processes instead of creating direct answers. Simulation modellers then take that distilled information, enrich it with expert knowledge on processes and create an agent-based model around the processes.
Decision-makers then apply the model to arrive at answers, instead of just what big data analysis gave us.
In this world, every business is forced to understand its processes, instead of relying on a data scientist to squeeze some correlations out of data. In this world, Data Science is a prerequisite for the next crucial step: simulation modelling.
Alright, enough dreaming. Analytics of Big Data is becoming huge and rightfully so. However, I argue that it should only be a first step for many business areas (such as operations, logistics, manufacturing). It should inform users about unknown business processes that feed into simulation models. Enriched with known processes, they can be employed for improved prediction and decision support. In that sense, Big Data Analytics and simulation modelling (including agent-based) can form an amazing tool for better business decisions.